
Section 3 presents the gas flow network data on an. (2007a) proposed the modied LASSO to select the signicant parameters of linear regression with autoregressive errors model. In Monte Carlo simulations, we illustrate the superiority of the proposed penalized estimation approach and argue that a combination of penalized and unpenalized estimation approaches results in overall best INAR model fits. Section 2 details the NNAR model and parameter estimation procedure using the profile least square method. the parameters in Poisson autoregressive model with sparse structure. In this paper, we study the Lasso estimator for fitting.
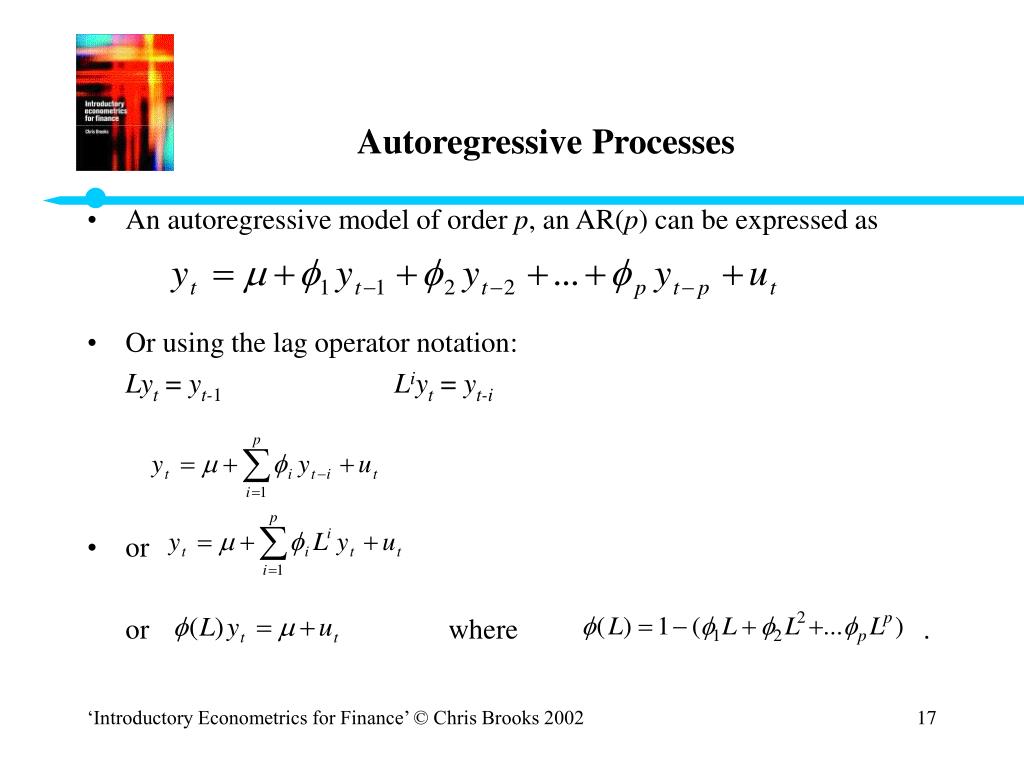
102, issue 3, 528-549 Abstract: The Lasso is a popular model selection and estimation procedure for linear models that enjoys nice theoretical properties. Journal of Multivariate Analysis, 2011, vol. For the data-driven selection of the penalization parameter, we propose two algorithms and evaluate their performance. Autoregressive process modeling via the Lasso procedure. Here, the sparsity of the AR model implies some of the autoregression coefficients are exactly zero, that must be excluded from the AR model. We adopt a double asymptotic framework where the maximal lag may increase with the sample size.
#Autoregressive process modeling via the lasso procedure series
In this paper, we study the Lasso estimator for tting au-toregressive time series models. This is the case, for example, in the frequently used INAR models with Poisson, negative binomially or geometrically distributed innovations. 13 Citations Metrics Abstract We study the adaptive least absolute shrinkage and selection operator (LASSO) for the sparse autoregressive model (AR). The Lasso is a popular model selection and estimation procedure for linear models that enjoys nice theoretical properties. Therefore, to improve the estimation accuracy, we propose a penalized version of the semiparametric estimation approach, which exploits the fact that the innovation distribution is often considered to be smooth, i.e. two consecutive entries of the PMF differ only slightly from each other. However, for small sample sizes, the estimation performance of this semiparametric estimation approach may be inferior. In this regard, a semiparametric estimation approach is a remarkable exception which allows for estimation of the INAR models without any parametric assumption on the innovation distribution. of the autoregressive matrix in a TvpVAR model.
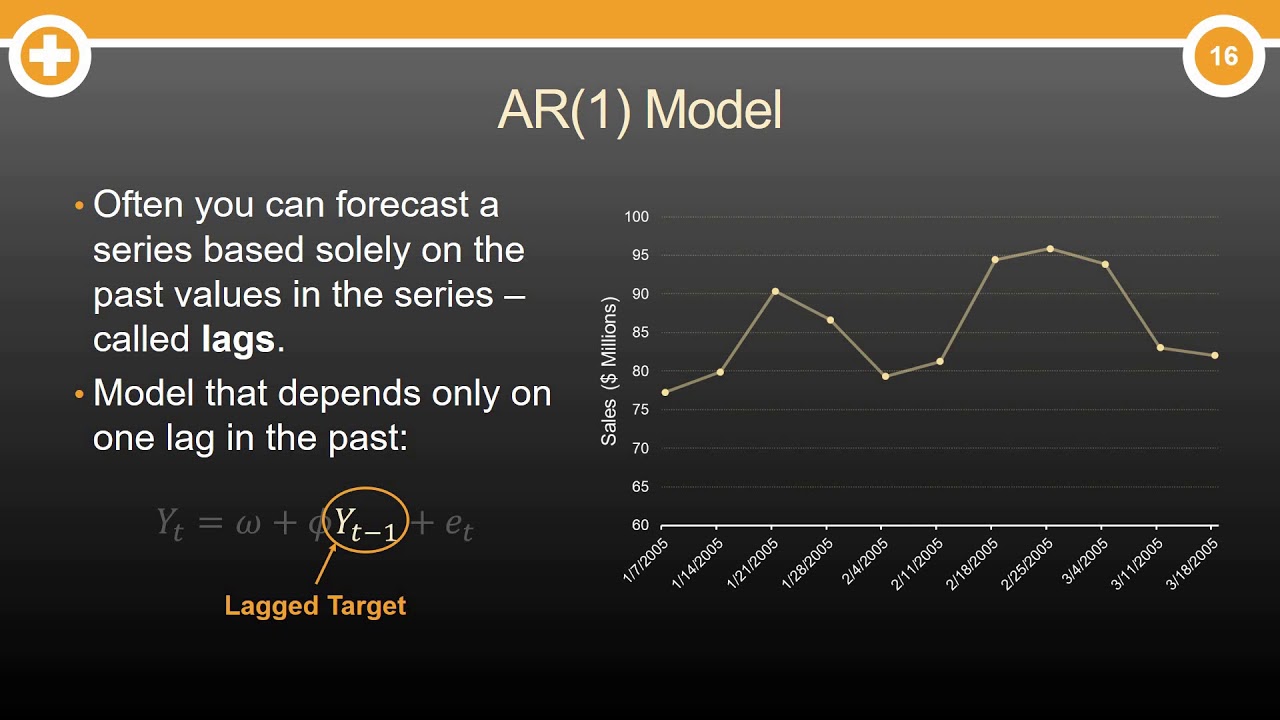
Popular models for time series of count data are integer-valued autoregressive (INAR) models, for which the literature mainly deals with parametric estimation. 5.1 Relative forecasting performance comparison across specific assets.
